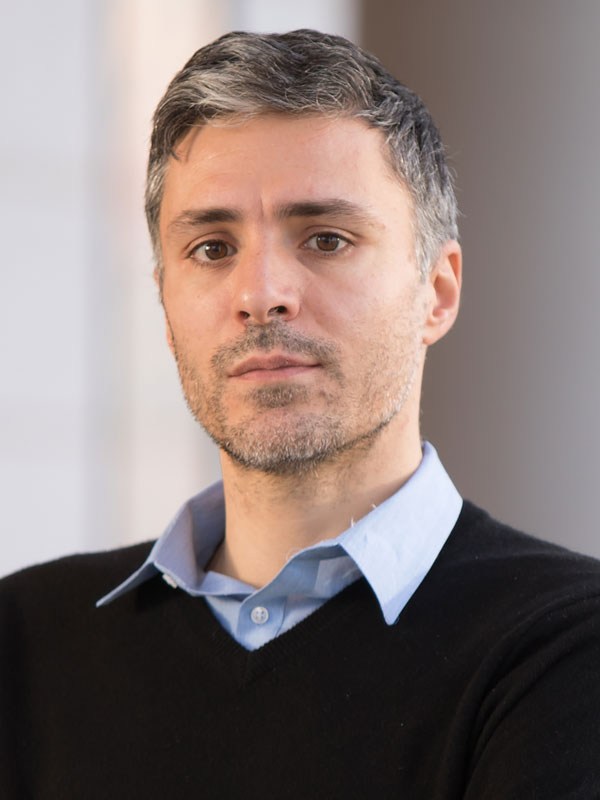
Ruben Coen-Cagli, Ph.D.
- Associate Professor, Department of Systems & Computational Biology
- Associate Professor, Dominick P. Purpura Department of Neuroscience
- Associate Professor, Department of Ophthalmology & Visual Sciences
Area of research
- We study neural computation to understand how the brain controls perceptual experience. Topics: neural coding and perception of natural stimuli; neural variability and perceptual uncertainty; information coding and transmission; image/video segmentation.
Phone
Location
- Albert Einstein College of Medicine Michael F. Price Center 1301 Morris Park Avenue 353B Bronx, NY 10461
Professional Interests
WHAT? The Coen-Cagli lab studies neural computation with the broader goal of explaining our perceptual experience. A central function of the visual system is to produce correct interpretations of sensory signals, to guide appropriate behavioral responses. However, the surrounding environment is in general ambiguous (e.g. different objects can produce similar retinal images) and computationally intractable (e.g. the same object can produce countless different retinal images). To solve these problems, the brain must estimate the probability of different interpretations of the sensory input. Understanding such probabilistic inference in natural sensory processing will be central to understanding perception, and much of the computation realized by cortical neurons.
HOW? The lab follows a hypothesis-driven approach to understanding cortical processing of natural stimuli and linking it to visual perception. Computer vision and machine learning provide insights into the complex structure of natural signals and how they could be processed efficiently. Probabilistic neural coding provides the theoretical framework to understand how veridical perception is achieved in face of abundant sensory noise and image ambiguities. We combine advances in both fields to generate novel hypotheses about cortical computation in natural vision, and test them experimentally with psychophysics in the lab and electrophysiology through collaborations.
WHY? Explaining how the human visual system achieves its impressive feats – from fast and accurate recognition of people and their actions, to the appreciation of Picasso’s Guernica – is a major goal of neuroscience, and more generally biology and medicine. Our research aims to contribute a substantial step forward to this endeavor, by taking a principled approach to studying the visual system in its natural operation mode. In the longer run, we hope this research will contribute to elucidating how the brain produces the vivid, coherent, stable percepts we experience in everyday life; to advancing technologies that could restore impaired vision and enhance normal vision; and to deciphering the neural basis of human visual creativity.
Selected Publications
(2024) M.A. Frechou, S.S. Martin, C.D McDermott, S. Gokhan, W. Tome, R. Coen-Cagli, T.J. Goncalves, Adult Neurogenesis improves spatial information encoding in the mouse hippocampus. Nature Communications 15:6410
(2024) R. Goris, R. Coen-Cagli, K.D. Miller, N.J. Priebe, M. Lengyel. Response sub-additivity and variability quenching in visual cortex. Nature Reviews Neuroscience: 25:237
(2023) J. Vacher,C. Launay, P. Mamassian, R. Coen-Cagli**. Measuring uncertainty in human visual segmentation PLoS Computational Biology 19(9): e1011483
(2023) O. Weiss, H.A. Bounds, H. Adesnik, R. Coen-Cagli. Modeling the diverse effects of divisive normalization on noise correlations. PLoS Computational Biology 19(11):e1011667
(2023) X. Pan, R. Coen-Cagli, O. Schwartz, Probing the Structure and Functional Properties of the Dropout-induced Correlated Variability in Convolutional Neural Networks. Neural Computation: 36(4):621
(2022) C. Launay, J. Vacher, R. Coen-Cagli. Unsupervised video segmentation algorithms based on flexibly regularized mixture models. 2022 IEEE ICIP 4073-4077
(2022) J. Vacher, C. Launay, R. Coen-Cagli. Flexibly regularized mixture models and application to image segmentation. Neural Networks 149:107.
(2022) J. Botsen, R. Coen-Cagli, A. Franklin, S, Solomon, M. Webster. Calibrating the visual system. Vision Research 201:108.
(2021) D. Festa, A. Aschner, A. Davila, A. Kohn, R. Coen-Cagli. Neuronal variability reflects probabilstic inference tuned to natural image statistics. Nature Communications 12:3635
(2021) S. Sokoloski, A. Aschner, R. Coen-Cagli. Modeling the neural code in large populations of correlated neurons. eLife 10:e64615
(2021) D. Herrera, L. Gomez-Sena, R. Coen-Cagli. Redundancy between spectral and higher-order statistics for natural image segmentation. Vision Research 187:55 (preprint: https://www.biorxiv.org/content/10.1101/2021.04.26.441524v1)
(2021) G. Dehaene,R. Coen-Cagli, A. Pouget. Investigating the representation of uncertainty in neuronal circuits. PLoS Computational Biology 17(1):e1008138.
(2021) D. Herrera, R. Coen-Cagli*, L. Gomez-Sena*. Flexible contextual modulation of naturalistic texture perception in peripheral vision. Journal of Vision 21(1):1(preprint: https://doi.org/10.1101/2020.01.24.918813)
(2020) J. Vacher, A. Davila, A. Kohn, R. Coen-Cagli. Texture interpolation for probing visual perception. NeurIPS 2020 spotight (preprint: ArXiv:2006.03698).
(2019) R. Coen-Cagli, S.S.. Solomon. Relating divisive normalization to neuronal response variability.Journal of Neuroscience 39(37):7344
(2016) A. Kohn, R. Coen-Cagli, I. Kanitscheider, A. Pouget, Correlations and neuronal population information. Annual Reviews of Neuroscience. 39:237-256.
(2015) I. Kanitscheider*, R. Coen-Cagli*, A. Pouget, The origin of information-limiting noise correlations. PNAS, 112(50): E6973-E6982
(2015) R. Coen-Cagli, A. Kohn*, O. Schwartz*, Flexible Gating of Contextual Modulation During Natural Vision. Nature Neuroscience, 18: 1648–1655
(2012) R. Coen-Cagli, P. Dayan, O. Schwartz, Cortical Surround Interactions and Perceptual Salience Via Natural Scene Statistics. PLoS Computational Biology, 8(3): e1002405