Where Innovation Meets Compassion
We achieve scientific excellence with innovative medical education and research that promotes community engagement and advances access to healthcare in the Bronx and beyond.
Explore Education
We combine humanistic care with research excellence to prepare medical students and graduate students in the biomedical sciences to become compassionate physicians and innovative scientific investigators. We’re proud to provide all M.D. students with the Ruth L. Gottesman Scholarship that covers the cost of tuition. Learn more about education at Einstein.
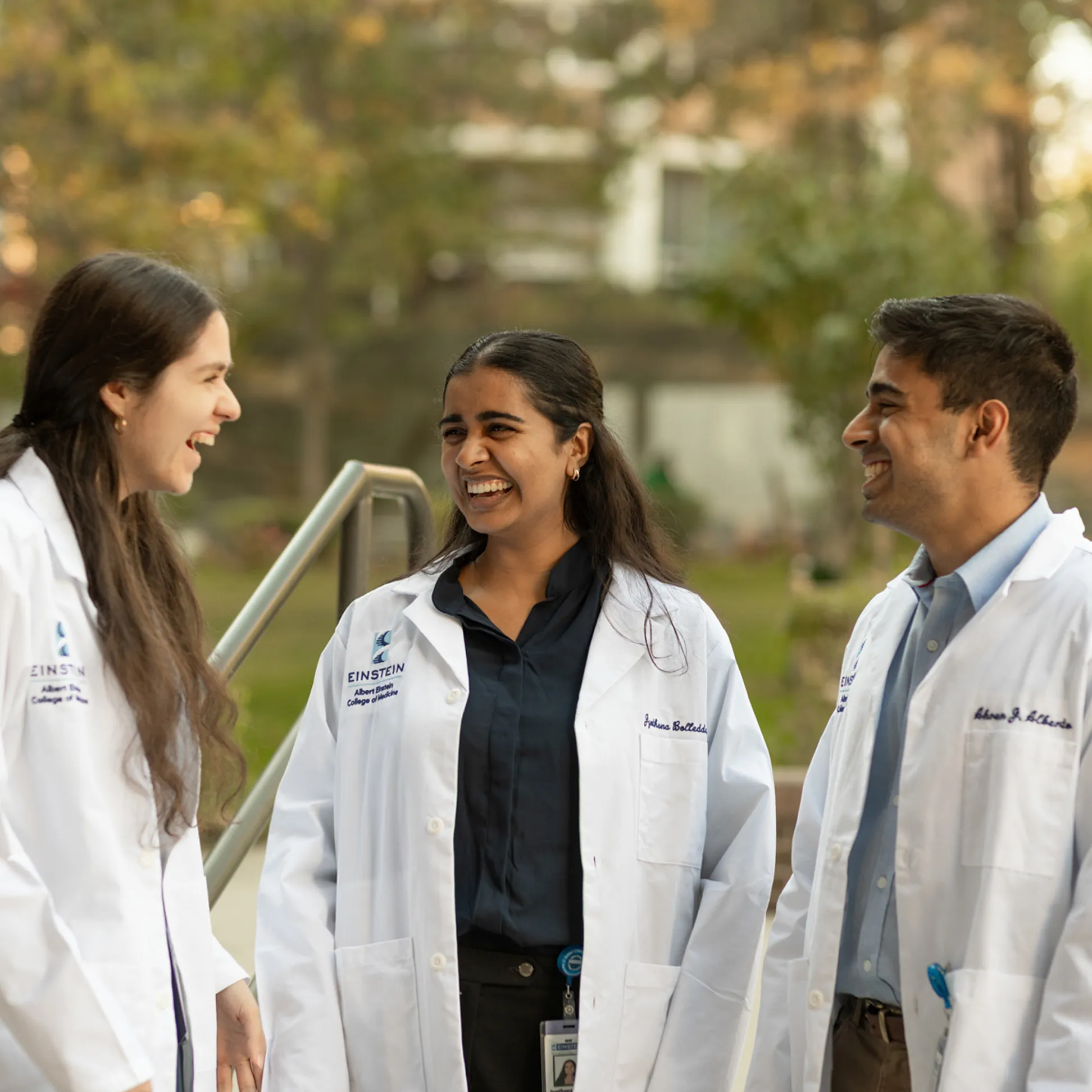
-
98%
match rate for M.D. Students in 2025
-
20%
of M.D. Students matched to an Einstein affiliate in 2025
-
1,000+
M.D., Ph.D., and M.D./Ph.D. students in 2025
-
2,000+
full-time faculty
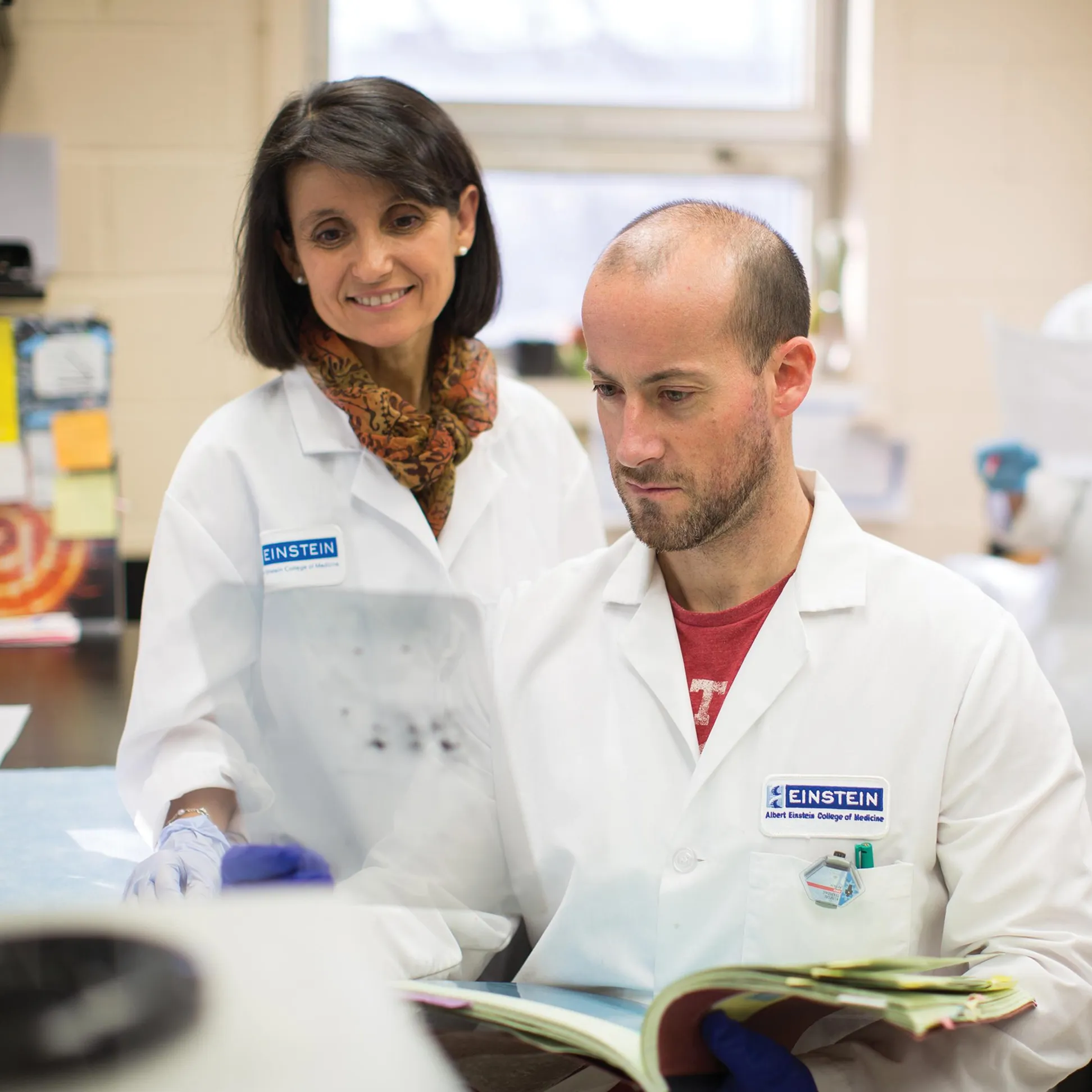
Ana Maria Cuervo, M.D., Ph.D., discoverer of chaperone-mediated autophagy, in the lab.
Explore Research
At Einstein’s many academic departments and centers of excellence, including several National Institutes of Health-designated research centers, we translate biomedical discoveries into effective new treatments, empowering clinicians to provide compassionate, patient-centered care informed by the latest biomedical research. Learn more about research at Einstein.
-
273M+
total research funding in 2024
-
3,211
published papers in 2024
-
20
research centers
-
36
global initiatives
Life at Einstein
We foster a culture of collaboration and accessibility that promotes innovation in education and biomedical research and advances health equity. Learn more about life at Einstein.
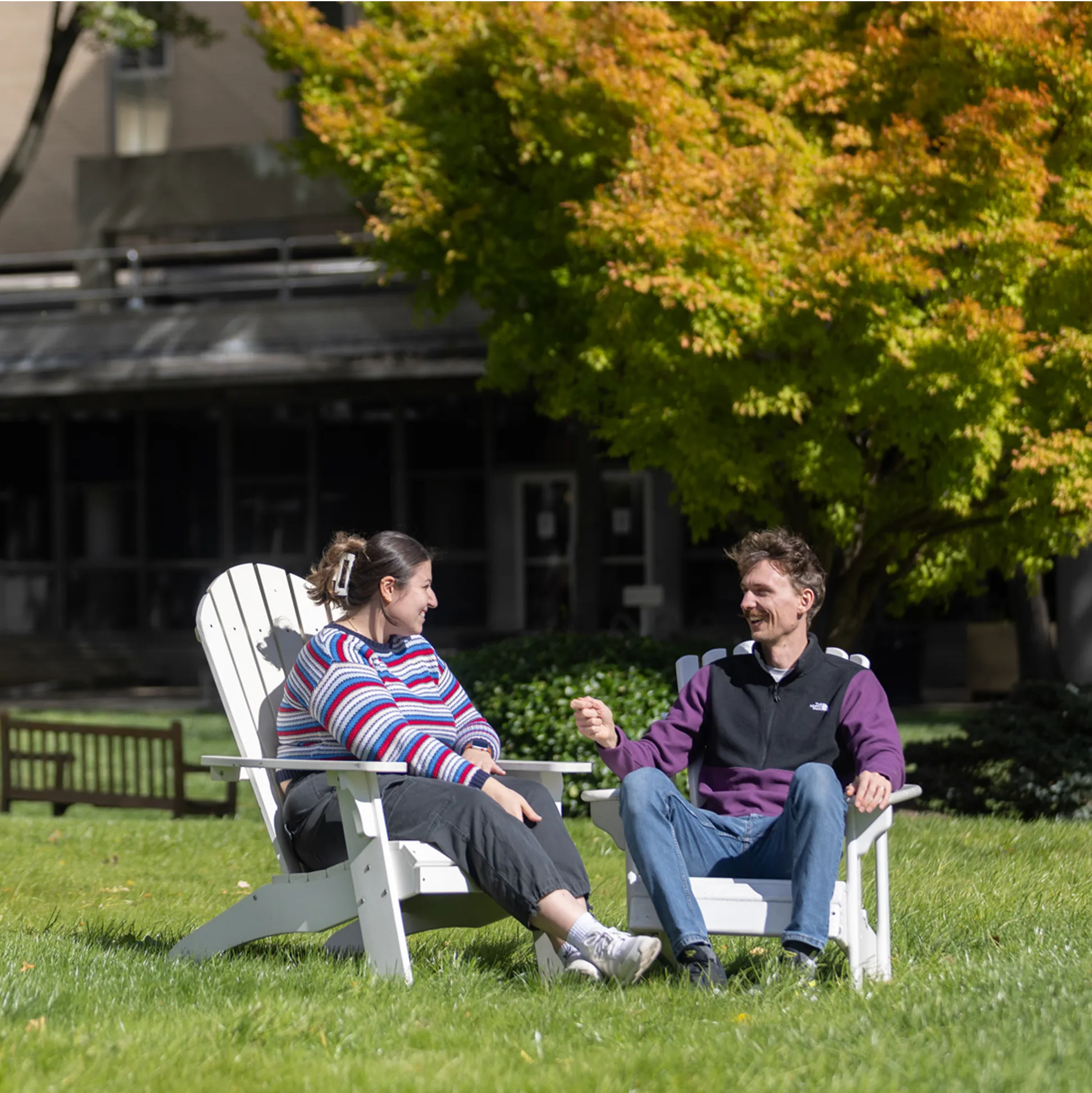
Events
Virtual
IP & Coffee Seminar Series: IP Strategies For Protecting Your Invention And Attracting Investment
Tuesday, July 29 from 01:00pm - 02:00pm
Monday, August 11 from 04:00pm - 05:30pm
Forchheimer Medical Science Building - 3rd Floor Lecture Hall